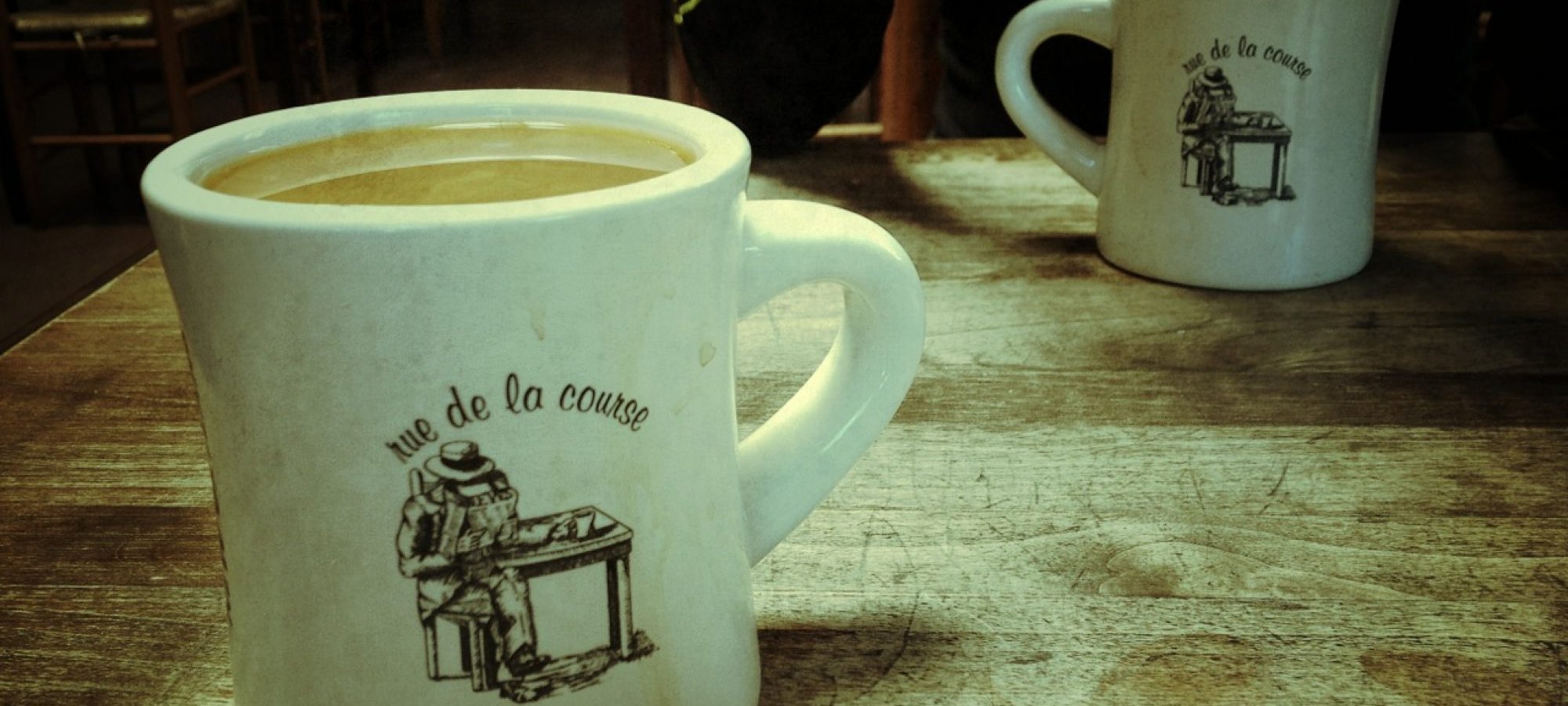
“They want, in short, to extract a logic from chance, which is like saying, blood from stones; and they are convinced they’ll succeed, today or at the latest, tomorrow”
Anomalous diffusion in biological fluids
The last twenty years have seen a revolution in tracking data of biological agents across an impressive range of spatial and temporal scales. An important and ubiquitous observation from these studies is that path trajectories of living organisms are often poorly described by the classical model for random movement in continuous time, Brownian motion.
To abuse a sentiment from Tolstoy: Brownian diffusions are all alike, but every anomalous diffusion is anomalous in its own way. In the details of the non-Brownian statistics lie vital clues to fundamental physical and physiological mechanisms of transport and interaction in living systems. The primary focus of my research in Applied Stochastic Processes has been to work directly with bioengineers and ecologists to determine the implications of anomalous diffusive data for both small scale physiology and for large scale animal population dynamics.
-Movement Ecology-
-Coral Ecology-
Sensing and decision-making in movement ecology
If it breathes and it moves, you can be assured that someone, somewhere out there has been tracking that animal and thinking deeply about how it interacts with its environment. Daily movement patterns of animal are quite random and it is tempting to model this behavior with Brownian motion, or simple discrete-time random walks. But these Markovian models ignore that if an animal takes a step in a certain direction, it probably had good reason to do so and it will continue in that direction.
Over the years I have collaborated with a number of ecologists to develop mathematical models that are tractable to analyze, but still respect this most basic fact about animal movement: organism sense their local environment and make decisions based on what is around them.
What’s new in the Stochastics Lab …
Toward statistical significance in topological data analysis
“My analysis has revealed a large hole in this point cloud. But is it possible that a hole of this size might arise due to randomness alone?”
Monte Carlo methods for PDE sensitivity
It has long been known that stochastic simulation methods can be more effective in computing the solutions of certain PDE models. But if we want to move the question to that of inference or optimization, we need to compute derivatives of our stochastic solutions. At first glance, it may not be clear what it means to differentiate a random variable with respect to a parameter, but in fact, probabilists have been doing this for years.